Artificial Intelligence (AI) and Machine Learning (ML) represent transformative technologies that have significantly reshaped the landscape of computing and decision-making. In this extensive exploration, we will delve into the fundamentals of AI and ML, understand their underlying principles, and examine real-world examples that illustrate their applications and impact on various industries.
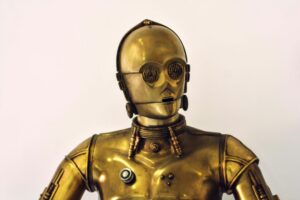
Understanding Artificial Intelligence (AI)
Defining AI: AI is a broad field of computer science dedicated to creating systems that exhibit human-like intelligence. The primary goal is to enable machines to perform tasks that traditionally require human cognitive abilities. AI systems are designed to learn, reason, problem-solve, and understand natural language.
Categories of AI: AI can be broadly classified into two categories: narrow AI (or weak AI) and general AI (or strong AI). Narrow AI is designed to perform specific tasks, such as image recognition or language translation, while general AI aims to possess human-like cognitive abilities across diverse domains.
Applications of AI: AI finds applications across various domains, including healthcare, finance, education, and entertainment. In healthcare, AI is utilized for disease diagnosis and drug discovery. In finance, it powers fraud detection and algorithmic trading. Educational platforms leverage AI for personalized learning experiences, and the entertainment industry uses AI for content recommendation and creation.
Navigating the World of Machine Learning (ML)
Understanding Machine Learning: Machine Learning is a subset of AI that focuses on creating algorithms capable of learning from data. Unlike traditional programming, where explicit rules are defined, ML systems learn patterns from data to improve their performance over time. ML can be categorized into supervised learning, unsupervised learning, and reinforcement learning.
Supervised Learning: Supervised learning involves training a model on a labeled dataset, where the algorithm learns to map input data to corresponding output labels. For example, in a spam email filter, the model is trained on labeled data containing examples of spam and non-spam emails.
Unsupervised Learning: Unsupervised learning deals with unlabeled data, aiming to uncover patterns and relationships within the dataset. Clustering and dimensionality reduction are common tasks in unsupervised learning. An example is clustering customer data to identify distinct segments.
Reinforcement Learning: Reinforcement learning involves an agent interacting with an environment and learning to make decisions based on feedback in the form of rewards or penalties. This paradigm is employed in scenarios like training a computer program to play chess or video games.
Real-world Examples of AI and ML Applications
1. Natural Language Processing (NLP): NLP is a branch of AI that focuses on the interaction between computers and human languages. An exemplary application is language translation services. Google Translate, powered by ML algorithms, learns from vast datasets to provide accurate translations across multiple languages.
2. Image and Speech Recognition: Image and speech recognition are integral parts of AI applications. Facial recognition technology, employed in security systems and social media, relies on ML algorithms to identify and verify individuals based on facial features. Similarly, voice assistants like Siri and Alexa utilize ML to comprehend and respond to spoken commands.
3. Healthcare Diagnostics: In healthcare, AI and ML play a pivotal role in diagnostics. For instance, IBM’s Watson for Oncology analyzes vast medical literature and clinical data to assist oncologists in identifying personalized treatment options for cancer patients.
4. Autonomous Vehicles: The development of autonomous vehicles heavily relies on AI and ML. These systems learn from diverse driving scenarios to make real-time decisions, enhancing safety and efficiency. Companies like Tesla use ML algorithms for advanced driver-assistance systems.
5. Recommender Systems: Recommender systems, prevalent in online platforms, leverage ML to analyze user behavior and preferences. Streaming services like Netflix use recommendation algorithms to suggest movies or shows based on a user’s viewing history.
6. Fraud Detection in Finance: ML is extensively employed in finance for fraud detection. Algorithms analyze transaction patterns and identify anomalies that could indicate fraudulent activities, providing a proactive approach to security.
The Synergy of AI and ML: Shaping the Future
1. Evolving Industries: The integration of AI and ML is reshaping traditional industries. In manufacturing, predictive maintenance powered by ML algorithms reduces downtime by anticipating equipment failures. AI-driven supply chain optimization enhances efficiency and reduces costs.
2. Personalized Learning in Education: AI and ML are revolutionizing education by enabling personalized learning experiences. Adaptive learning platforms use algorithms to tailor educational content based on individual student progress and learning styles, fostering a more effective and engaging educational environment.
3. Precision Agriculture: In agriculture, AI and ML contribute to precision farming. Drones equipped with ML algorithms analyze crop health, enabling farmers to make data-driven decisions about irrigation, fertilization, and pest control.
4. Healthcare Revolution: AI and ML have the potential to revolutionize healthcare by accelerating drug discovery, improving diagnostics, and enhancing patient care. Personalized medicine, guided by genetic data and AI analysis, promises more effective and targeted treatment plans.
5. Environmental Monitoring: Environmental monitoring benefits from AI and ML applications. ML algorithms analyze data from various sources, including satellites and sensors, to track climate changes, deforestation, and other environmental factors, aiding in conservation efforts.
Conclusion: Shaping Tomorrow with AI and ML
In conclusion, Artificial Intelligence and Machine Learning represent the forefront of technological innovation, ushering in a new era of possibilities across diverse industries. From revolutionizing healthcare and finance to enabling personalized learning and enhancing environmental sustainability, the impact of AI and ML is profound.
As we navigate the ever-evolving landscape of AI and ML, it becomes imperative to understand their capabilities, ethical considerations, and potential societal impacts. The synergy between human intelligence and machine learning algorithms holds the promise of addressing complex challenges and shaping a future where intelligent machines contribute to human progress. The journey into the realms of AI and ML continues to unfold, with each innovation bringing us closer to a world where intelligent systems work seamlessly to augment our capabilities and improve the quality of life.
Navigating the Digital Frontier: A Glimpse into the Tapestry of Technology
One thought on “1. Introduction to AI and ML: A Comprehensive Exploration”